QRS peak detection#
Detection of cardiac cycles from ECG signals
# setup
import sys
import numpy as np
import scipy.signal as sp
from matplotlib import pyplot as plt
import wfdb
Download records#
Identify and download records in the MIMIC III Waveform Database
# Select the first record
selected_record = '3000063_0013'
database_name = 'mimic3wdb/1.0/30/3000063/'
print("Selected record: {}".format(selected_record))
# load data from this record
start_seconds = 20
no_seconds_to_load = 10
fs = 125
record_data = wfdb.rdrecord(record_name = selected_record, sampfrom = fs*start_seconds, sampto = fs*(start_seconds + no_seconds_to_load), pn_dir = database_name)
print("{} seconds of data loaded from: {}".format(no_seconds_to_load, selected_record))
# Plot the data loaded from this record
title_text = "First record from " + database_name + " (record: " + selected_record + ")"
wfdb.plot_wfdb(record=record_data, title=title_text, time_units='seconds')
Selected record: 3000063_0013
10 seconds of data loaded from: 3000063_0013
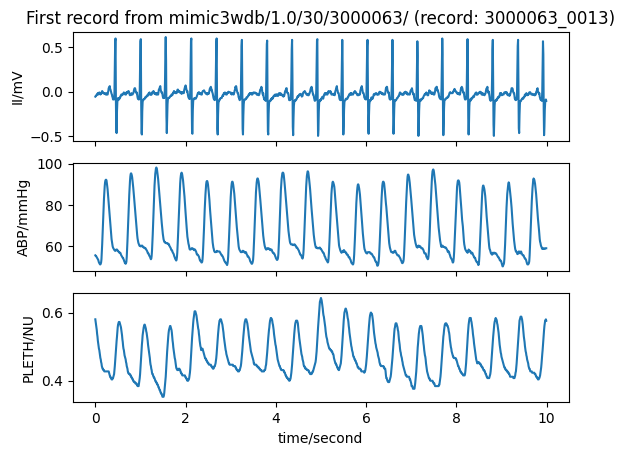
Separate records#
Separate ECG, PPG and ABP signals
# ECG, PPG and ABP extraction
h = 0
for i in record_data.sig_name:
if i == 'II':
print('ECG at position ' + str(h))
ecg = record_data.p_signal[:,h]
elif i == 'PLETH':
print('PPG at position ' + str(h))
ppg = record_data.p_signal[:,h]
elif i == 'ABP':
print('ABP at position ' + str(h))
abp = record_data.p_signal[:,h]
else:
print('Other signal (' + i + ') at position ' + str(h))
h = h + 1
t = np.arange(start_seconds,(start_seconds + no_seconds_to_load),1.0/fs)
fig, (ax1,ax2,ax3) = plt.subplots(3, 1, sharex = True, sharey = False)
ax1.plot(t, ecg)
ax1.set(xlabel = '', ylabel = 'ECG')
ax2.plot(t, ppg)
ax2.set(xlabel = '', ylabel = 'PPG')
ax3.plot(t, abp)
ax3.set(xlabel = 'Time [s]', ylabel = 'ABP')
ECG at position 0
ABP at position 1
PPG at position 2
[Text(0.5, 0, 'Time [s]'), Text(0, 0.5, 'ABP')]
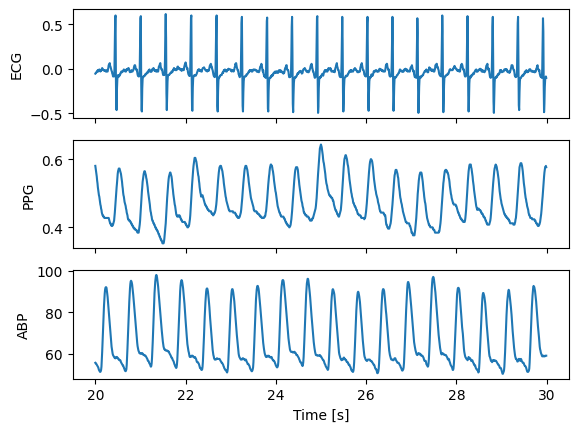
# Remove NaNs
x, = np.where(np.isnan(ecg))
if len(x) != 0:
print('NaNs in ECG: ' + str(x))
for i in x:
ecg[i] = ecg[i - 1]
x, = np.where(np.isnan(ppg))
if len(x) != 0:
print('NaNs in PPG: ' + str(x))
for i in x:
ppg[i] = ppg[i - 1]
x, = np.where(np.isnan(abp))
if len(x) != 0:
print('NaNs in ABP: ' + str(x))
for i in x:
abp[i] = abp[i - 1]
Filter data#
# Filter ECG
sos_ecg = sp.butter(10, [0.5, 40], btype = 'bp', analog = False, output = 'sos', fs = fs)
ecg_ff = sp.sosfiltfilt(sos_ecg, ecg)
# Filter PPG
sos_ppg = sp.butter(10, [0.2, 10], btype = 'bp', analog = False, output = 'sos', fs = fs)
ppg_ff = sp.sosfiltfilt(sos_ppg, ppg)
# Filter ABP
sos_abp = sp.butter(10, 10, btype = 'low', analog = False, output = 'sos', fs = fs)
abp_ff = sp.sosfiltfilt(sos_abp, abp)
fig, (ax1,ax2,ax3) = plt.subplots(3, 1, sharex = False, sharey = False)
fig.suptitle('Filtered signals')
ax1.plot(t, ecg, color = 'red')
ax1.plot(t, ecg_ff, color = 'orange')
ax1.set_xlabel('Time [s]')
ax1.set_ylabel('ECG [V]')
ax2.plot(t, ppg, color = 'red')
ax2.plot(t, ppg_ff, color = 'orange')
ax2.set_xlabel('Time [s]')
ax2.set_ylabel('PPG [V]')
ax3.plot(t, abp, color = 'red')
ax3.plot(t, abp_ff, color = 'orange')
ax3.set_xlabel('Time [s]')
ax3.set_ylabel('ABP [V]')
plt.subplots_adjust(left = 0.1,
bottom = 0.1,
right = 0.9,
top = 0.9,
wspace = 0.4,
hspace = 0.4)
#plt.show()
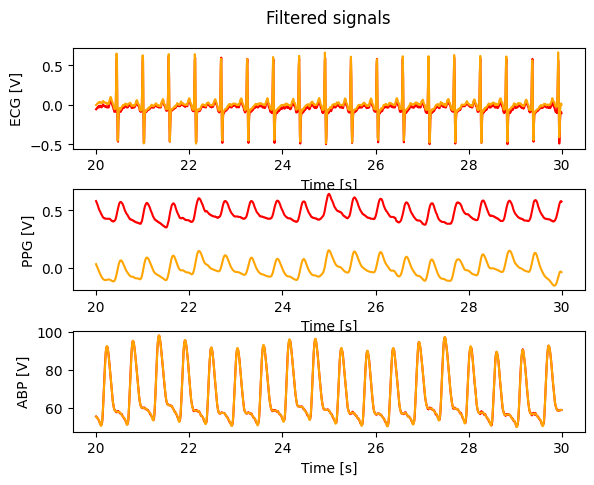
QRS detection#
Define QRS detector functions#
# QRS detection function
def qrs_detect(ecg,fs,w):
"""
Description: QRS peak detection and correction
Inputs: ecg, array with ECG signal [user defined units]
fs, sampling rate of signal [Hz]
w, window length for analysis [s]
Outputs: qrs, positions of R peaks [number of samples]
n_int, number of intervals of length w in the signal
Libraries: NumPy (as np), SciPy (Signal, as sp), Matplotlib (PyPlot, as plt)
Version: 1.0 - June 2022
Developed by: Elisa Mejía-Mejía
City, University of London
"""
# Normalization of ECG
ecg_n = (ecg - np.min(ecg))/(np.max(ecg) - np.min(ecg))
ecg_n = ecg_n - np.mean(ecg_n)
# Peak detection in windows of length w
n_int = np.floor(len(ecg)/(w*fs))
for i in range(int(n_int)):
start = i*fs*w
stop = (i + 1)*fs*w - 1
#print('Start: ' + str(start) + ', stop: ' + str(stop))
aux = ecg_n[range(start,stop)]
locs, amps, nlocs = rpeakdetect(aux,fs,0.2)
locs = locs + start
if i == 0:
qrs = locs
else:
qrs = np.append(qrs,locs)
if n_int*fs*w != len(ecg_n):
start = stop + 1
stop = len(ecg_n)
aux = ecg_n[range(start,stop)]
if len(aux) > 20:
locs, amps, nlocs = rpeakdetect(aux,fs,0.2)
locs = locs + start
qrs = np.append(qrs,locs)
# Peak correction
# (1) Not peaks
med_rr = np.median(np.diff(qrs))
med_amp = np.median(ecg[qrs])
pos_for = 0
pos_rev = 0
for i in range(len(qrs)):
cur_amp = ecg[qrs[i]]
if i == 1: # Peak is at the first position of the signal
next_amp = ecg[qrs[i] + 1]
cond = cur_amp >= next_amp
#print(cur_amp, next_amp, cond)
elif i == len(ecg): # Peak is at the last position of the signal
prev_amp = ecg[qrs[i] - 1]
cond = cur_amp >= prev_amp
#print(cur_amp, prev_amp, cond)
else:
next_amp = ecg[qrs[i] + 1]
prev_amp = ecg[qrs[i] - 1]
cond = cur_amp >= prev_amp and cur_amp >= next_amp
#print(cur_amp, prev_amp, next_amp, cond)
if not(cond):
# Forward search
j = qrs[i] + 1
if i == len(qrs) - 1:
stop = len(ecg)
else:
stop = qrs[i + 1]
while j < stop:
cur_amp = ecg[j]
next_amp = ecg[j + 1]
prev_amp = ecg[j - 1]
if not(cur_amp > next_amp and cur_amp > prev_amp):
j = j + 1
else:
if ecg[j] >= 0.5*med_amp:
pos_for = j
j = stop
else:
j = j + 1
# Reverse search
j = qrs[i] - 1
if i == 0:
stop = 1
else:
stop = qrs[i - 1]
while j > stop:
cur_amp = ecg[j]
next_amp = ecg[j + 1]
prev_amp = ecg[j - 1]
if not(cur_amp > next_amp and cur_amp > prev_amp):
j = j - 1
else:
if ecg[j] >= 0.5*med_amp:
pos_rev = j
j = stop
else:
j = j - 1
# Selection of peak
if pos_for != 0 and pos_rev != 0:
pos = [pos_for, pos_rev]
if i - 1 == 0:
dif = - pos
else:
dif = qrs[i - 1] - pos
dif_med = np.abs(dif - med_rr)
#print(dif_med)
min_dif = np.min(dif_med)
min_dif, = np.where(dif_med == min_dif)
#print(min_dif,pos(min_ind))
qrs[i] = pos(min_ind)
# (2) Low peaks
med_amp = np.median(ecg[qrs])
#print(len(qrs))
result, = np.where(ecg[qrs] < 0.5*med_amp)
if len(result) > 0:
#print(result)
qrs = np.delete(np.array(qrs),result)
#print(len(qrs))
# (3) Too close or too far
rr = np.diff(qrs)
avg_rr = np.mean(rr)
med_rr = np.median(rr)
q3_rr, q1_rr = np.percentile(rr,[75, 25])
iqr_rr = q3_rr - q1_rr
#print(rr, avg_rr, med_rr, q3_rr, q1_rr, iqr_rr)
if (med_rr < 0.5*fs or avg_rr < 0.5*fs) and (iqr_rr > 0.2*fs):
result, = np.where(rr <= med_rr)
qrs = np.delete(qrs, result)
med_rr = np.median(rr)
# (a) Too close
ind, = np.where(rr < 0.5*med_rr)
if len(ind) != 0:
i = 1
while i <= len(ind):
ind = no.sort(np.append(ind, ind[i] + 1))
#print(ind)
i = i + 2
qrs = np.delete(qrs, ind)
# (b) Too far
rr = np.diff(qrs)
ind, = np.where(rr > 1.5*med_rr)
th_amp = 0.5*np.median(ecg[qrs])
#print(ind, th_amp)
if len(ind) != 0:
i = 0
while len(ind) != 0:
#ind = np.round(ind)
#qrs = np.round(qrs)
#print(i, ind[i], len(qrs))
if qrs[ind[i]] == qrs[-1]:
aux = ecg[qrs[ind[i]]:len(ecg)]
else:
aux = ecg[qrs[ind[i]]:qrs[ind[i] + 1]]
locs, _ = sp.find_peaks(aux)
ind_del, = np.where(aux[locs] < th_amp)
locs = np.delete(locs, ind_del)
if len(locs) == 0:
new = med_rr + qrs[ind[i]] - 1
elif len(locs) == 1:
new = locs + qrs[ind[i]] - 1
else:
aux_dif = np.abs(locs - med_rr)
min_dif = np.min(aux_dif)
ind_new, = np.where(aux_dif == min_dif)
new = locs[ind_new] + qrs[ind[i]] - 1
qrs = np.sort(np.append(qrs, new))
rr = np.diff(qrs)
ind, = np.where(rr > 1.5*med_rr)
rr = np.diff(qrs)
ind_del, = np.where(rr < 0.3*fs)
rr = np.delete(rr, ind_del)
#fig = plt.figure()
#plt.plot(ecg, color = 'black')
#plt.scatter(qrs,ecg[qrs],marker = 'o',color = 'red')
return qrs, n_int
# R peak detect
def rpeakdetect(ecg, fs, th):
"""
Description: QRS peak detection based on rpeakdetect2.m by G. Clifford
A batch QRS detector based upon that of Pan, Hamilton and Tompkins:
J. Pan & W. Tompkins. A real-time QRS detection algorithm. IEEE Trans Biomed Eng, vol. BME-32 NO. 3. 1985.
P. Hamilton & W. Tompkins. Quantitative Investigation of QRS Detection Rules Using the MIT/BIH Arrythmia
Database. IEEE Trans Biomed Eng, vol. BME-33, NO. 12.1986.
Inputs: ecg, array with ECG signal [user defined units]
fs, sampling rate of signal [Hz]
th, threshold for peaks in integrated waveform - Default: 0.2
Outputs: locs, positions of R peaks [number of samples]
amps, amplitudes of R peaks [user defined units]
nlocs, number of R peaks found
Libraries: NumPy (as np), SciPy (Signal, as sp), Matplotlib (PyPlot, as plt)
Version: 1.0 - June 2022
Developed by: Elisa Mejía-Mejía
City, University of London
"""
# Create time array
t = np.divide(range(0,len(ecg) - 1), fs)
# Preprocessing:
# (1) Filter data
sos = sp.butter(6, 40, btype = 'low', analog = False, output = 'sos', fs = fs)
ecg_f = sp.sosfiltfilt(sos, ecg)
# (2) Differentiate ECG
ecg_d = np.diff(ecg_f)
# (3) Square ECG
ecg_s = ecg_d*ecg_d
# (4) Integrate data
if fs >= 256:
d = np.ones(int(np.round(7*fs/256)))
else:
d = np.ones(21)
ecg_fir = sp.lfilter(d, 1, ecg_s)
ecg_med = sp.medfilt(ecg_fir, kernel_size = 9)
# Remove filter delay:
delay = np.ceil(len(d)/2)
ecg_med = ecg_med[int(delay):len(ecg_med)]
# Find peaks:
# (1) Find highest bumps in data
start_int = round((len(ecg) - 1)/4)
stop_int = round(3*(len(ecg) - 1)/4)
max_h = np.max(ecg_med[start_int:stop_int])
# (2) Determine left and right boundaries
ecg_med = np.insert(ecg_med,0,0)
ecg_med = np.append(ecg_med,0)
n_left = 0
n_right = 0
for i in range(len(ecg_med)):
if i > 0 and i < len(ecg_med):
if ecg_med[i] > (th*max_h) and ecg_med[i - 1] < (th*max_h): # left boundary
if n_left == 0:
left_bound = i - 1
n_left = 1
else:
left_bound = np.append(left_bound,i - 1)
if ecg_med[i] > (th*max_h) and ecg_med[i + 1] < (th*max_h): # right boundary
if n_right == 0:
right_bound = i - 1
n_right = 1
else:
right_bound = np.append(right_bound,i - 1)
#print(left_bound)
#print(right_bound)
# (4) Look through all possibilities
if left_bound[0] > right_bound[0]:
right_bound = np.delete(right_bound,0)
if left_bound[-1] > right_bound[-1]:
left_bound = np.delete(left_bound,0)
nlocs = 0
for i in range(len(left_bound)):
#print(i)
lb = left_bound[i]
rb, = np.where(np.array(right_bound) > lb)
if len(rb) != 0:
rb = right_bound[rb[0]]
#print(lb, rb)
amp = np.max(ecg[lb:rb])
pos, = np.where(np.array(ecg[lb:rb]) == np.amax(ecg[lb:rb]))
pos = pos[0]
pos = int(pos + lb)
#print(pos, amp)
if nlocs == 0:
locs = pos
amps = ecg[pos]
else:
locs = np.append(locs, pos)
amps = np.append(amps, ecg[pos])
nlocs = nlocs + 1
#print(locs)
#fig = plt.figure()
#plt.plot(ecg)
#plt.plot(ecg_f)
#plt.plot(ecg_d)
#plt.plot(ecg_s)
#plt.plot(ecg_fir)
#plt.plot(ecg_med)
#plt.scatter(locs, amps, marker = 'o')
return locs, amps, nlocs,
# Detect cardiac cycles
qrs, n_int = qrs_detect(ecg_ff,fs,5)
fig = plt.figure()
plt.title('QRS detection')
plt.plot(t, ecg_ff, color = 'black')
plt.scatter(start_seconds + qrs/fs, ecg_ff[qrs], color = 'red', marker = 'o')
<matplotlib.collections.PathCollection at 0x7fb7ad332950>
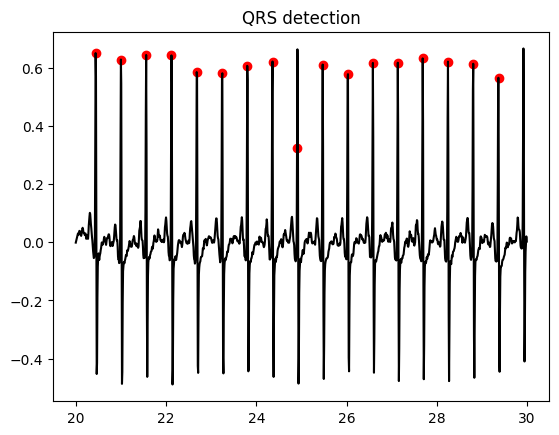